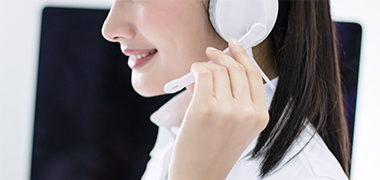
- Contact:Minister Xu
- Mobile:13841408476
- Tel:024-44837288
- Fax:024-44837004
- E-mail:xuming58@126.com
- Web:m.wanderlustamericana.com
- Address:No.110 XiangHuai Road
Benxi Economic Development Zone
Liaoning Province
SKF Machine learning-based predictive maintenance changes the game
03 Nov,2020
SKF’s AutoML-based offering combines machine process data with vibration and temperature data to reduce costs and enable new business models.
Automated Machine Learning, AutoML, is enabling a completely new way for machine and factory operators to approach performance and machine output. At the center of this development is the combined expertise resulting from SKF and an Israeli start-up which was acquired by the Swedish bearing manufacturer in 2019.
Automated Machine Learning (AutoML) applies pretrained Machine Learning algorithms to real-time process data to identify anomalous patterns and warn technicians of evolving asset failure. AI is responsible for choosing which machine learning models are applied and maintaining these models over time while they run in production. This capability enables quicker modelling and higher accuracy.
Eitan Vesely, SKF AI Offering Manager, says: “What’s really exciting about this development is that we are able to combine asset vibration data with temperature and other types of process data generated by the asset. Essentially, 2+2=5 in terms of extrapolating value from the combined data set and what it means in terms of actionable insights.”
“For customers, this means earlier failure alerts and insights that provide maintenance technicians with th e time and information they need to plan maintenance and establish a diagnosis, before a machine breaks down.”
AutoML supports new business models
On its own, AutoML-based predictive maintenance is a powerful tool for anticipating failure and gaining a thorough understanding of asset health at the sensor, asset and plant levels. What it means in terms of business models is something altogether different. The AutoML solution from SKF - SKF Enlight AI – enables the implementation of outcome-based business models, where customers pay a fixed fee for a combined offer from SKF. This can include bearings, sensors, lubrication, seals and remanufacturing.
With SKF Enlight AI in place, and the decision-making knowledge it creates, the customer and SKF can work together to improve the performance of the machine, both from an output and sustainability point of view.
“With the knowledge and understanding of a machine’s performance that AutoML enables, we can work with the customer to plan maintenance and optimize inventories of spare parts in a completely new way. Simply put, avoiding unplanned downtime is a significant gain for everyone involved,” explains Vesely.
SKF Enlight AI in action: A pulp and paper use case
A major pulp and paper company in Latin America wanted to pilot the SKF Enlight AI solution on a set of critical assets: motor pumps operating the pre-bleaching system, crucial machines for the entire production process.
Due to recurring unexpected failures, the pumps were becoming a production bottleneck, causing annual losses of hundreds of thousands of dollars. The interdependencies between the pumps forced the entire pre-bleaching system offline every time a pump failed.
The mill urgently needed to reduce unscheduled downtime of the pumps and their growing impact on annual downtime costs. To this end, the mill wanted to receive early failure predictions in an easy-to-use interface and attain greater visibility into asset process data.
Typically, AI applies pretrained Machine Learning algorithms to real-time sensor data to identify evolving asset failure. In this case, the customer wanted to evaluate the solution’s effectiveness by testing it out on process data from existing failures.
Two pump failures were used to assess SKF Enlight AI’s capabilities. The first pump, Pump A, had an oil leak detected during a visual inspection on 26 December. The leak was determined non-critical and a planned shutdown was scheduled for the end of January. Operational losses for this planned shutdown amounted to $150,000.
Pump B failed unexpectedly on 31 December, two days after vibration analysis had first detected an evolving fault. Root cause analysis revealed that a bearing failure was responsible for the shutdown. In total, the operational cost of Pump B’s unscheduled downtime was $250,000.
These failures dramatically increased maintenance costs and disrupted routine work orders over the course of several weeks. However, SKF Enlight AI proved that this situation could have been alleviated using machine learning-based predictive maintenance.
SKF Enlight AI detected anomalous asset behavior in the historical data of both pumps from mid-December. Based on the time to failure estimations provided for each pump, maintenance could have scheduled the planned maintenance for Pump A for an earlier date, before the estimated failure of Pump B. This would have left sufficient time to schedule the necessary resources and spare parts and execute a planned shutdown at the end of December to fix problems in both pumps.
In this way the total loss, which was about $400,000, would have been only $150,000, the smaller value of the two shutdowns. In the best-case scenario, the potential savings would have been $250,000.
Eitan Vesely explains: “During this evaluation process, both vibration and process data were analyzed, and the conclusion was clear: using both data sources, more failures were predicted than if only one data source would have been used.”
“Based on the value demonstrated in this pilot project we are now in process to roll out the solution across hundreds of assets in three different plants”